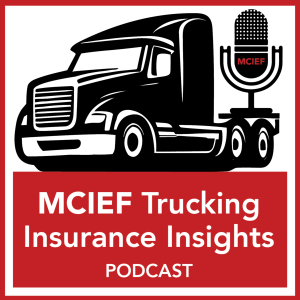
Saturday Apr 05, 2025
April Truck Stop Podcast: Beyond the Black Box: Demystifying AI in Trucking Insurance with Cover Whale
Artificial intelligence (AI) and machine learning (ML) are no longer just buzzwords—they’re actively reshaping the landscape of commercial trucking insurance. In a recent conversation with industry leaders Jim Schoonover, John Love, Nyles Oppenheimer (Chief Revenue Officer at Cover Whale), and Darryl Siry (CIO and COO at Cover Whale), the group explored how AI is driving innovation and delivering measurable results in efficiency, underwriting, and risk assessment.
Where AI Is Making the Biggest Impact
Nyles Oppenheimer identified four key pillars of AI application in insurance:
-
Telematics and ELD data
-
Risk scoring and pricing
-
Claims processing automation
-
Underwriting speed
While the hype around generative AI like ChatGPT continues to grow, Darryl Siry reminded us that insurance has always relied on data to assess risk. What’s changing is the scale and speed at which we can now process that data—thanks to cloud computing and modern AI tools.
From Static to Continuous Underwriting
One of the biggest shifts happening in the industry is the move from traditional underwriting to continuous underwriting. Rather than assessing risk once a year, insurers can now monitor real-time driver behavior through telematics, enabling them to:
-
Detect deteriorating or improving risk profiles
-
Adjust pricing dynamically
-
Reward safe drivers with incentives
It’s a proactive approach that not only benefits insurers but also promotes safer driving habits across the board.
Better Risk Assessment for Motor Carriers
Insurance costs remain a significant concern for motor carriers. AI tools are helping by making risk assessments more granular and accurate. By identifying high-risk drivers early, insurers can offer more favorable pricing to low-risk fleets—helping good actors thrive.
John Love emphasized that while the core questions of risk assessment haven't changed, AI allows insurers to answer them more precisely and shift from passive to active underwriting.
Collaboration and Transparency Are Key
The panel also underscored the need for openness between carriers, agents, and policyholders. Explaining how data is collected, analyzed, and used to inform decisions builds trust and encourages smarter risk management across the board.
Navigating the Ethical and Regulatory Landscape
Darryl Siry addressed the regulatory challenges that come with AI, particularly the concern over “black box” models. He noted the distinction between:
-
Predictive models (transparent and explainable)
-
Generative AI models (less explainable)
However, he believes the commercial trucking sector is better positioned than most due to the objective nature of the data being used (e.g., speed, braking, GPS patterns), which helps reduce concerns about bias and discrimination.
Looking Forward: What’s Next for AI in Insurance?
As AI adoption accelerates, insurers face both opportunities and challenges. Siry highlighted the internal resistance to change in large insurance companies, contrasting it with the agility of MGAs and InsureTechs like Cover Whale.
John Love concluded by stressing the importance of pairing automation with deep industry expertise, predicting a future where objective, data-driven models help insurers serve a broader spectrum of risks efficiently.
No comments yet. Be the first to say something!